The Intricacies of the Machine Learning Process
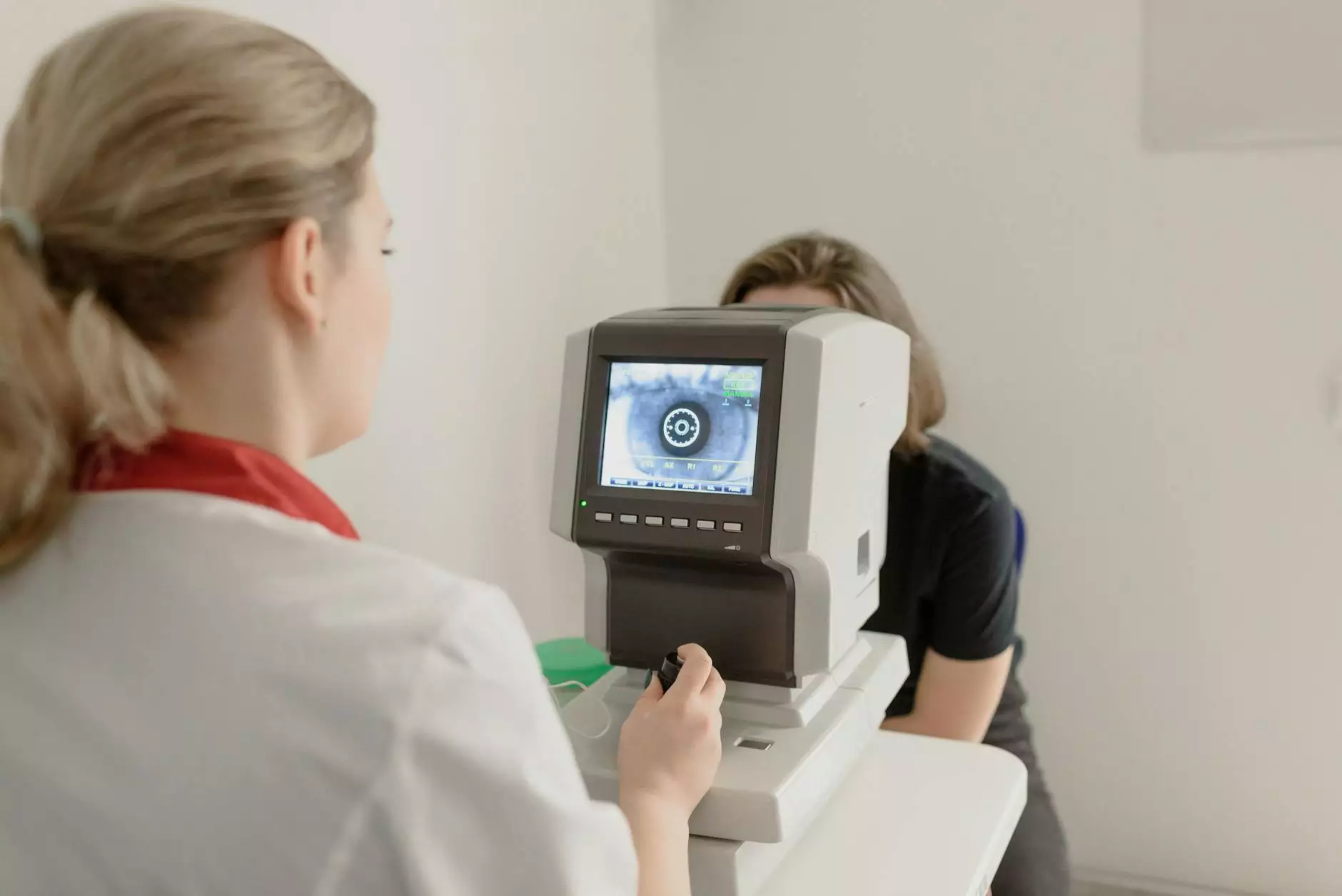
Machine learning has revolutionized the way businesses operate in the digital age. Understanding the machine learning process is crucial for harnessing its power and gaining a competitive edge in various industries.
What is Machine Learning?
Machine learning is a subset of artificial intelligence that enables systems to learn and improve from experience without being explicitly programmed. It involves the development of algorithms that allow computers to analyze and interpret large amounts of data to identify patterns and make informed decisions.
Key Steps in the Machine Learning Process
- Data Collection and Preparation: The first step in the machine learning process involves gathering relevant data and cleaning it to remove inconsistencies and errors.
- Feature Engineering: This step involves selecting and extracting relevant features from the data to enhance the performance of the machine learning model.
- Model Selection: Choosing the appropriate algorithm or model that best suits the data and the problem being solved.
- Training the Model: Training the selected model on the data to learn patterns and make accurate predictions.
- Evaluation and Optimization: Assessing the performance of the model, making necessary adjustments, and optimizing it for better results.
- Deployment: Finally, deploying the trained model into a production environment for making real-time predictions.
Applications of Machine Learning
Machine learning is widely used across various industries, including healthcare, finance, e-commerce, and marketing. Some common applications of machine learning include:
- Personalized Recommendations: E-commerce platforms use machine learning algorithms to recommend products based on user preferences and browsing history.
- Fraud Detection: Financial institutions utilize machine learning to detect and prevent fraudulent activities by analyzing transaction patterns.
- Medical Diagnosis: Healthcare providers leverage machine learning to diagnose diseases and predict patient outcomes based on clinical data.
- Customer Segmentation: Marketing teams use machine learning to segment customers based on behavior and preferences for targeted campaigns.
Challenges in the Machine Learning Process
Despite its numerous benefits, the machine learning process comes with its own set of challenges. Some common challenges include:
- Data Quality: The success of machine learning models heavily relies on the quality and quantity of data available for training.
- Overfitting: Overfitting occurs when a model performs well on training data but fails to generalize to unseen data.
- Interpretability: Understanding and interpreting the decisions made by complex machine learning models can be challenging for stakeholders.
- Scalability: Scaling machine learning systems to handle large volumes of data efficiently can be a daunting task.
Conclusion
Understanding the machine learning process is essential for leveraging the power of AI to drive innovation and growth in businesses. By following the key steps and overcoming challenges, organizations can harness the potential of machine learning to make informed decisions and stay ahead of the competition.
explain about machine learning process